Let’s talk about salads… Do people still get sick from contaminated leafy greens?
Last year, the Canadian Public Health Agency officially asked consumers to avoid romaine lettuce until their investigation of some serious illness incidents was completed.
During the same period, a dedicated U.S. Food and Drug Administration (FDA) 2020 Leafy Greens Action Plan was deployed, utilizing sophisticated technologies such as blockchain and machine learning to ensure that people don’t get sick from salad greens.
A couple of weeks ago, colleagues from Wageningen Food Safety Research and other European institutes shared that they will include green vegetables on the list of priorities for an upcoming EU initiative that will apply AI to big data to detect emerging food risks, like biological hazards.
So, what on earth is going on with our green salads?
A Food Safety Tech article by Deane Falcone gives an excellent introduction to the problem. According to Deane, certain characteristics make popular leafy green vegetables (like lettuce or spinach) an easy target for bacteria, such as Escherichia coli (E. coli). This is due to a wide array of reasons, including:
- They are produced over wider areas, making it difficult to track contamination.
- They grow low to the ground, exposing the edible, leafy parts to contaminated water.
- Unlike other vegetables, lettuce and other leafy greens are less often cooked prior to consumption.
- Green leaf characteristics make it easier for bacteria to attach, becoming more resistant to removal by washing.
A CBC News story tried to explain in simple terms what caused foodborne illnesses throughout North America. CBC highlighted that between 2009 and 2018, the U.S. FDA and Centers for Disease Control and Prevention (CDC) say they identified 40 foodborne outbreaks of infections from this strain of E. coli in the U.S. with a confirmed or suspected link to leafy greens. The FDA says it is working to increase its understanding of how leafy greens become contaminated, and recent FDA reports suggest that cows near produce fields or water sources could be part of the problem.
Very interesting that one of the suspicious romaine lettuces came from a Santa Barbara (California) farm that may have had a contaminated water source. After investigating the incident, the FDA noted the “extensive wild animal activity” and cited that adjacent land use by nearby cattle and horses “may have had the potential to be reservoirs of E. coli O157: H7.”
This brings two questions to mind:
- Did similar lettuce safety problems appear in other parts of the world?
- Could one have predicted (and hopefully prevented) these food safety incidents?
I went to FOODAKAI for answers.
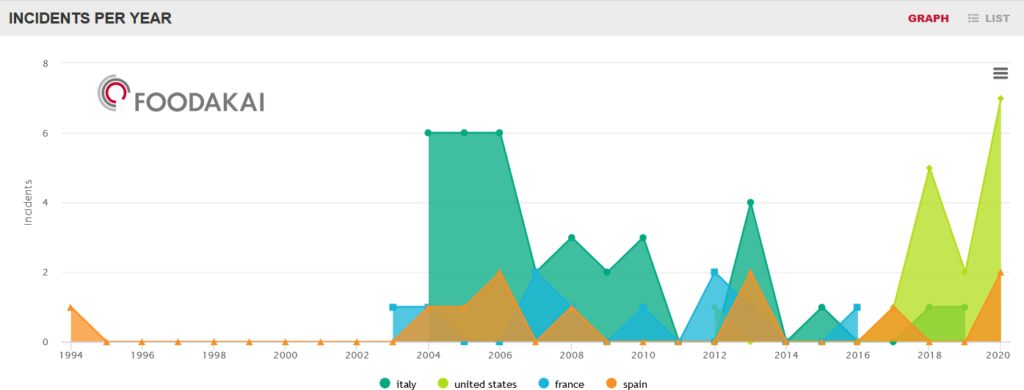
Lettuce incidents during the past 25 years in the 4 most exposed countries (source FOODAKAI)
First, I investigated the countries that have reported the most leafy green incidents over the past 20 years. Digging into data about food safety incidents in Italy, France, and Spain, I found that the majority of incidents were due to the existence of unauthorized pesticide residues. The U.S. case is different: there was a spike in food recalls over the past couple of years, but the majority were due to E. coli, not unauthorized pesticides.
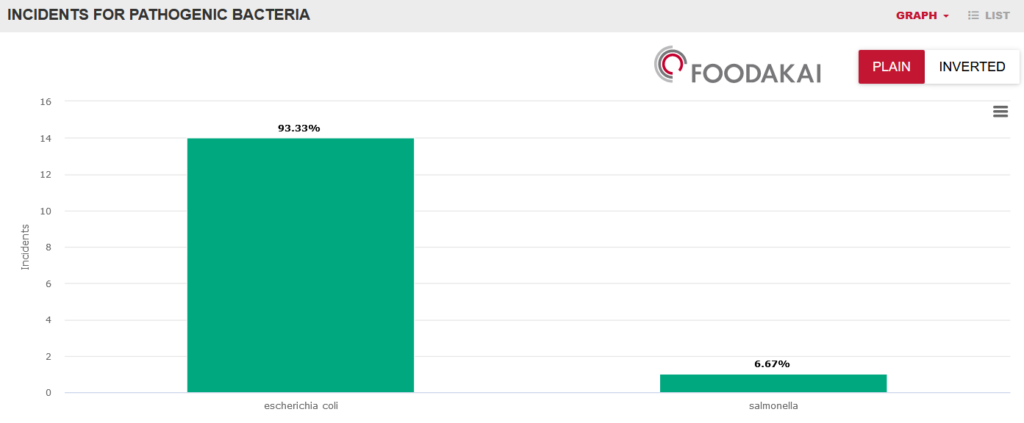
Biological hazards responsible for US lettuce incidents (source: FOODAKAI)
Then, I tried to understand if the right AI model could help us avoid such E. coli outbreaks.
As the Food Safety Tech article states, E. coli incidents will likely remain prevalent because of the challenge of testing all irrigation water for large and widespread production fields. Once microbial contaminants are present on fresh leafy produce, their complete removal by washing cannot be guaranteed, and it is very difficult to monitor every plot of crops continuously.
Based on our experience, there would be two ways of using predictive analytics in this case.
One is focused on using AI models that help initiate a rapid response to an emerging risk before its impact scales. Appropriate prediction algorithms (such as time-series) can immediately identify a rapid increase in illness or recall incidents anywhere in the world. FOODAKAI’s Global Predictions Dashboard offers such a functionality, by flagging sudden increases in incidents that cannot be justified by seasonality or other variables in the historical data.
We would pay special attention to issues with a forecasted rapid escalation over the following months. These are the early signals that tell us appropriate preventive measures need to be taken. For example, the predictions for lettuce incidents during the next 12 months seem to be positive – still, the overall food safety incidents in salads are expected to be high. We are actually seeing a 21% increasing tendency for food safety incidents in salads for the next 6 months. As for lettuce, the risk evolution suggests that a 35% increase in incidents from E. coli is predicted for the next 11 months.
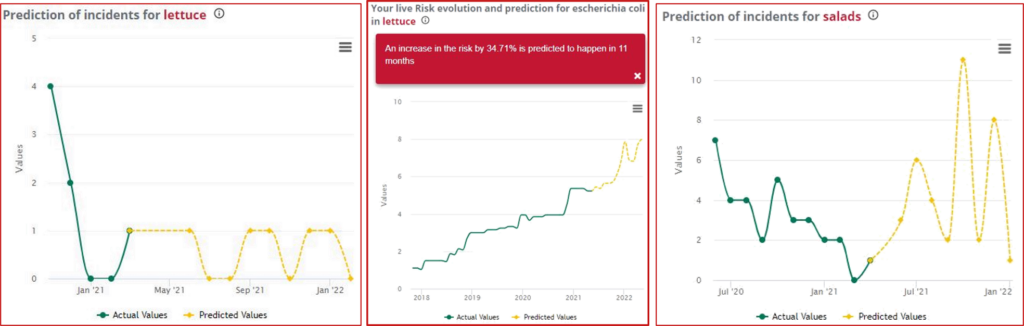
Predictions for Lettuce & Salads (source FOODAKAI)
The other way to take advantage of predictive analytics is by putting AI models in place that can provide mid- to long-term prediction of risks. Hans Marvin and Yamine Bouzembrak work on a systems’ approach for longer-term hazard forecasting. Their initial experimental results indicate that incorporating macro-variables in the model, such as climate conditions and agrochemical use, may predict food contamination levels with high accuracy. Such approaches can help foresee and prevent risks influenced by environmental and other factors – e.g., location of lettuce farms, consumption of agrochemical products in the area, and climate conditions.
Both approaches are in line with the FDA’s Action Plan, in which machine learning and traceback investigation tools aim to leverage quantitative food risk assessment to further support risk mitigation strategies.
In our view, it is important to look at such predictive solutions, because of the operational decision-making capabilities that they offer. We should be looking beyond one-off prediction experiments that may reveal the root causes of foodborne illness.
I believe that the real value of such tools lies in their application to real-life decision-making settings. They are the digital crystal balls that will help us anticipate, and hopefully prevent, undesired risks on a daily basis.
If you’d like to discover how FOODAKAI can help your Food Safety & Quality team prevent food recalls by monitoring & predicting risks, schedule a call with us!