When we refer to predictive analytics for the food safety sector there are plenty of factors that we should take into account in order to generate trustworthy results. First of all, we should consider the business problem which is to be solved through predictions. Then much thought should be put into selecting the right data and the right algorithms. However, having done all the data work, the next step is to focus on the right prediction metrics.
In this episode of our mini video series, Giannis Stoitsis, CTO, and partner at Agroknow, explains how we can measure if a prediction algorithm is working properly.
There are so many metrics that one can use which makes it very important to choose the right ones for the prediction problem at hand. You will hear terms like recall, precision, confusion matrix, mean absolute error, mean squared error, root mean square error, f1-score and others. But what do all these metrics really mean?
Ideally, the selection of the evaluation metrics should be linked to the formulation of the problem at hand. It should also reflect the real settings in which the algorithm is expected to operate.
Let’s take a look at an example in which we are more interested in coverage than accuracy. Which ingredient categories will face increased recalls and border rejections in 2020 compared to 2019.
To monitor the accuracy of the predictions in this experiment we used the Absolute Mean Percentage Error, by comparing the actual numerical value for 2019 with the ones predicted by the model using the historical values until the end of 2018.
Having an inaccurate prediction was not critical in our scenario: we were more interested in uncovering the trends. On the other hand, good coverage was critical. Not being able to calculate a prediction for one or more product categories would mean that we would have no clue about what to expect during the next 12 months.
Stay tuned for our next video!
If you’d like to discover how FOODAKAI can help your Food Safety & Quality team prevent product recalls by monitoring & predicting risks, schedule a call with us!
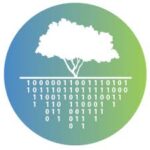